In the realm of IoT technology, terms like Artificial Intelligence (AI) and Machine Learning (ML) often get tossed around interchangeably, leading to confusion among enthusiasts and professionals alike. While they are interconnected, there are distinct differences between the two that are essential to grasp for anyone delving into the world of tech.
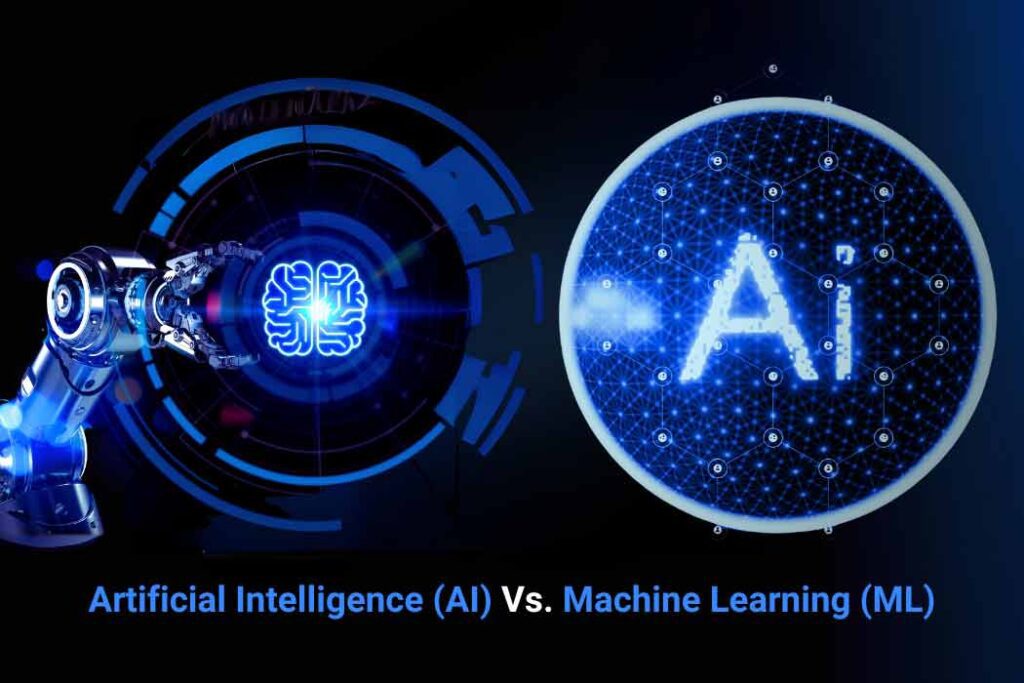
In this comprehensive guide, we’ll unravel the intricacies of AI and ML, clarifying their roles, functionalities, and impact on the ever-evolving landscape of the Internet of Things or IoT Technology.
What is Artificial Intelligence (AI)?
The term artificial intelligence, or AI, describes the mimicking of cognitive processes like learning, problem-solving, and decision-making in machines by means of software. With exposure to data, artificial intelligence (AI) systems can learn and improve their performance over time, in contrast to traditional computer programs that follow set instructions.
The Essence of AI in IoT
Within the framework of the Internet of Things (IoT), AI plays a pivotal role in enhancing connectivity and efficiency across diverse devices and systems. By leveraging advanced algorithms and data analytics, AI-enabled IoT applications can intelligently interpret and respond to complex data streams, facilitating automation, predictive maintenance, and personalized user experiences.
AI in IoT finds applications across various domains, including healthcare, finance, Fleet and transportation, manufacturing, and entertainment. Examples include self-driving cars, fraud detection systems, recommendation algorithms used by streaming services, and virtual personal assistants like Siri and Alexa.
Exploring Machine Learning (ML)
Conversely, machine learning is a branch of artificial intelligence that concentrates on creating algorithms that let computers see patterns in data and make judgments based on that data without the need for explicit programming. Essentially, ML algorithms iteratively learn from data, identifying patterns and trends to generate insights or predictions.
ML’s Impact on IoT Advancements
In the context of IoT or Internet of things, Machine Learning algorithms empower devices and sensors to extract valuable insights from massive datasets generated by interconnected systems. By harnessing ML capabilities, IoT solutions across Industries can optimize resource utilization, detect anomalies, and enhance predictive analytics for informed decision-making.
ML algorithms come in a variety of forms, such as semi-supervised, unsupervised, reinforcement, and supervised learning. Each type serves a specific purpose, such as classification, regression, clustering, or reinforcement of decision-making.
Real-world Examples of AI and ML
In the real world, AI and ML are deployed in numerous applications across different industries, revolutionizing how tasks are performed and decisions are made.
AI Applications
– Virtual Personal Assistants: Siri, Alexa, Google Assistant
– Autonomous Vehicles: Tesla Autopilot, Waymo
– Healthcare: Medical diagnosis, drug discovery
– Finance: Fraud detection, algorithmic trading
ML Applications
– Recommendation Systems: Netflix, Amazon
– Natural Language Processing: Chatbots, language translation
– Image Recognition: Facial recognition, object detection
– Predictive Analytics: Customer churn prediction, demand forecasting
Key Differences: AI vs. ML
Scope and Functionality – While AI encompasses a broader spectrum of technologies aimed at replicating human intelligence, Machine Learning specifically focuses on algorithms that enable computers to learn from data and improve performance over time.
Dependency on Data – AI systems may or may not rely solely on data-driven learning; they can incorporate predefined rules or symbolic reasoning. In contrast, Machine Learning heavily depends on data for training models and making predictions or decisions.
Flexibility and Adaptability – AI systems demonstrate varying degrees of flexibility, ranging from rule-based systems to deep learning models capable of autonomously acquiring new knowledge. ML algorithms, however, are inherently adaptable, continuously refining their predictions based on new data inputs.
Implications for IoT Integration – In the context of IoT integration, understanding the distinctions between AI and ML is crucial for designing scalable, efficient, and intelligent systems. While AI provides the overarching framework for cognitive capabilities, ML serves as the engine driving data-driven insights and optimizations within IoT ecosystems.
Conclusion
While Artificial Intelligence (AI) and Machine Learning (ML) are often used interchangeably, they represent distinct paradigms within the broader landscape of IoT technology.
By delineating their functionalities, dependencies, and implications for IoT integration, organizations and developers, Legacy IoT can harness their synergies to unlock unprecedented opportunities for innovation and advancement in the digital age.
As the Internet of Things continues to permeate diverse sectors, the synergy between AI and ML will undoubtedly redefine the boundaries of possibility, propelling us towards a Future Role of IoT characterized by unprecedented connectivity, efficiency, and intelligence.